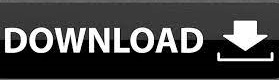

You can practice these projects and enhance your data science skills. Original text- ProjectPro offers 200+ solved end-to-end Data Science and Big Data reusable project solutions. Here’s an example of how extractive text summarization works. which are implemented using the Python libraries Gensim or Sumy. The extractive summarization method works with the help of algorithms such as LexRank, Luhn, LSA, etc. This technique extracts the required text according to the specified criteria without making significant changes to the documents. Extractive summarization uses a scoring mechanism to rank the relevance of phrases to select just those that are most relevant to the source document's meaning. The extractive text summarization approach involves extracting essential words from an original document and combining them to create a summary. There are mainly two different text summarization approaches- extractive and abstraction. Human summarizers are more biased and inaccurate than automatic summarizing techniques.īusiness providers can enhance the volume of texts they can handle by using automatic or semi-automatic summarizing systems. The text summarization technique boosts the volume of data stored in a particular space. Text summarization shortens reading time.Īutomatic summarization improves the effectiveness of searching, i.e., it speeds up searching for necessary information among vast quantities of data. Here are some significant reasons why we need text summarization. As a result, we need methods for automatic text summarization.
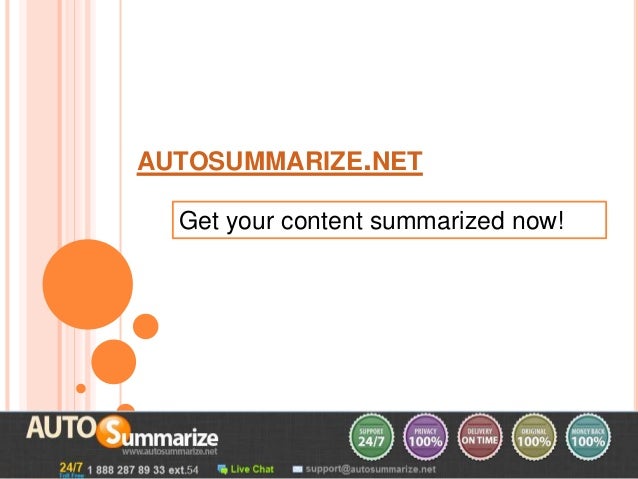
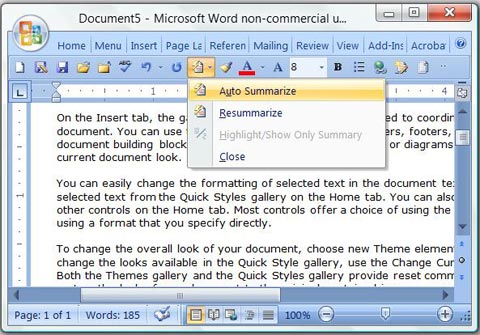
Manually summarizing vast amounts of textual data is a challenging task that can lead to inefficient outcomes. MLOps Project to Build Search Relevancy Algorithm with SBERT View Project Let us further look into the key reasons behind the growing demand for Text Summarization. Text summarization is beneficial for several NLP tasks, including text classification, legal text summaries, news summaries, generating headlines, etc. This is precisely where text summarization comes into the picture! There is a high demand for machine learning algorithms that can quickly summarize lengthy texts and offer accurate insights. Most of this text data needs to be minimized to more straightforward, concise summaries containing essential details to browse and analyze them more easily. As per Statista, by 2025, the total amount of data created, recorded, copied, and consumed worldwide is expected to exceed 180 zettabytes. The language of the summary should be concise and straightforward so that it conveys the meaning to the reader. Text summarization in NLP is the process of creating summaries from large volumes of data while maintaining significant informational elements and content value. This is why we need text summarization as it aids in shortening lengthy texts. Furthermore, with the rising number of articles being produced and the digitization of printed media articles, keeping track of the growing number of web articles has become extremely difficult. With the rising digital era and its impact on the publishing industry, investing time to read an article mindfully is no longer an option, given time constraints. Text summarization in Natural Language Processing (NLP) certainly significantly impacts human life.
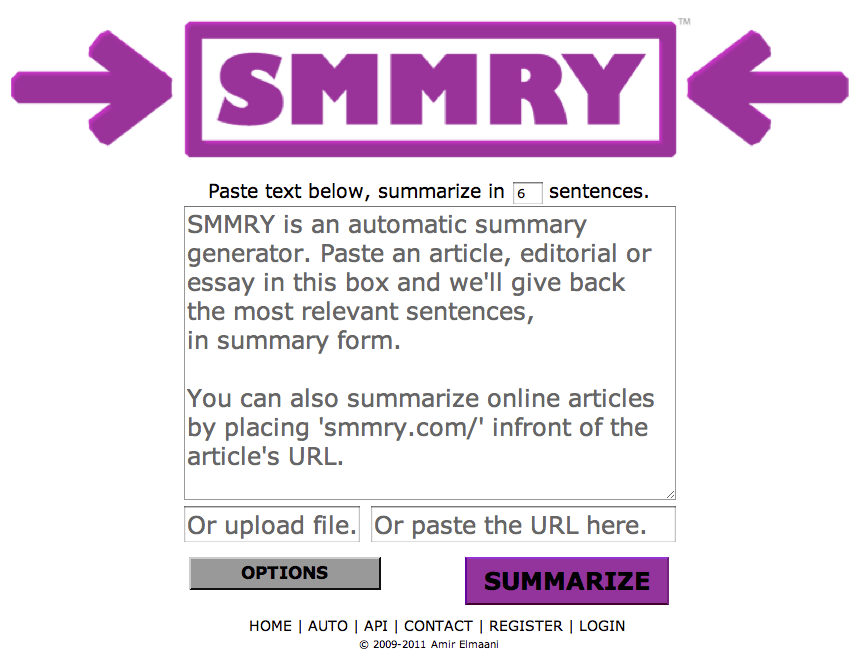
Top Machine Learning Text Summarization Algorithms.
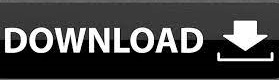